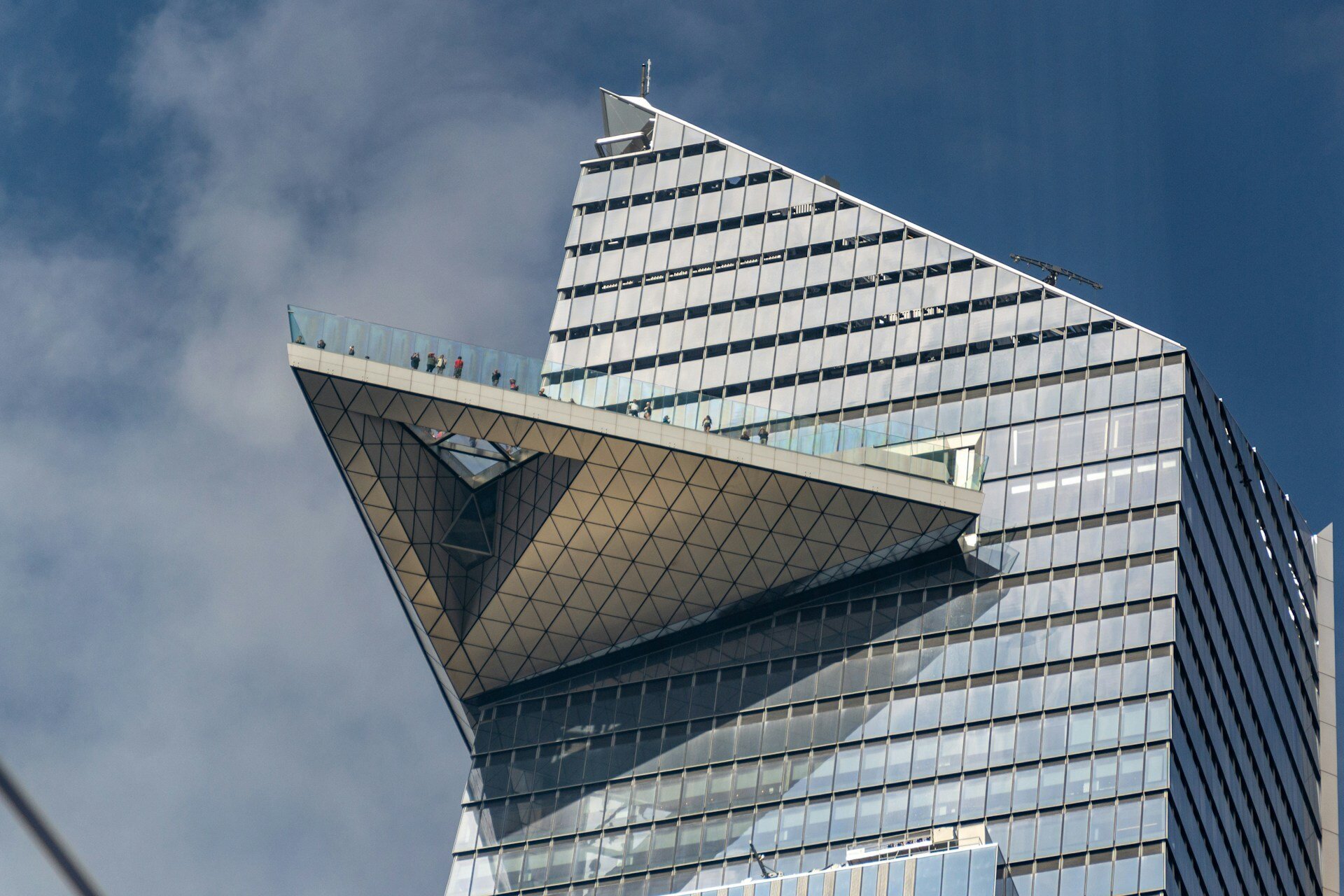
In recent years, artificial intelligence (AI) has experienced a surge in adoption across various industries, from healthcare to automotive, finance to entertainment. The transformative potential of AI is founded on its ability to process vast amounts of data, learn from it, and make decisions.
That said, the scalability of AI-enabled applications also poses challenges especially concerning latency, security, and cost. To address these challenges, the integration of edge computing has emerged as a crucial strategy. By processing data closer to its source, edge computing offers a powerful framework for scaling AI-enabled applications efficiently and effectively.
Understanding Edge Computing
Edge computing refers to the practice of processing data near the data source, such as IoT devices, sensors, or local servers, rather than relying on centralized cloud data centers. This paradigm shift aims to reduce latency, minimize bandwidth usage, and enhance data privacy and security.
In the context of AI, edge computing enables real-time data processing and decision-making. This is vital for applications requiring immediate responses, such as autonomous vehicles, smart grids, and industrial automation.
Advantages of Edge Computing for AI Scalability
- Reduced Latency: AI applications often require real-time or near-real-time data processing to function optimally. Cloud-based AI solutions can suffer from latency due to the distance between the data source and the cloud servers. Edge computing mitigates this issue by bringing the processing power closer to the data source, enabling faster response times. This is particularly important for applications like autonomous vehicles, where split-second decisions can mean the difference between safety and disaster.
- Bandwidth Efficiency: Transmitting vast amounts of data to and from the cloud can be costly and inefficient. Edge computing reduces the need to send raw data to central servers by processing it locally. Only the essential insights or processed data are sent to the cloud, significantly reducing bandwidth usage and associated costs. This is especially beneficial in environments with limited or expensive network connectivity.
- Enhanced Security and Privacy: Data privacy and security are paramount concerns in AI applications, particularly in sectors like healthcare and finance. Edge computing enhances security by keeping sensitive data closer to its source and reducing the amount of data transmitted over networks. Localized processing minimizes the risk of data breaches during transmission and complies with stringent data protection regulations.
- Scalability and Resilience: Edge computing facilitates the scalable deployment of AI applications by distributing the processing load across multiple edge devices. This decentralized approach not only enhances scalability but also ensures system resilience. In case of a network failure or cloud service outage, edge devices can continue to operate independently, maintaining the functionality of critical applications.
Challenges and Solutions in Integrating Edge Computing with AI
Despite its advantages, integrating edge computing with AI presents several challenges. One significant challenge is the limited computational power and storage capacity of edge devices compared to centralized cloud servers. To address this, efficient AI models and algorithms tailored for edge devices are being developed. Techniques such as model quantization, pruning, and federated learning enable the deployment of AI models on resource-constrained devices without compromising performance.
Another challenge is the complexity of managing a distributed network of edge devices. Ensuring seamless coordination, updates, and maintenance across numerous devices can be daunting. Solutions such as edge orchestration platforms and centralized management systems help streamline these processes, providing a unified framework for deploying, monitoring, and managing edge AI applications.
Use Cases and Applications
- Smart Cities: Edge computing powers AI applications in smart cities by processing data from sensors and cameras locally. For instance, traffic management systems use edge AI to analyze real-time traffic data, optimize signal timings, and reduce congestion without relying on cloud-based processing.
- Healthcare: In healthcare, edge computing enables real-time patient monitoring and diagnostics. Wearable devices equipped with AI algorithms can analyze vital signs on the edge, alerting healthcare providers to potential issues immediately, thereby improving patient outcomes.
- Industrial Automation: Manufacturing facilities utilize edge computing to implement AI-driven predictive maintenance. Edge devices analyze data from machinery to predict failures and schedule maintenance proactively, minimizing downtime and enhancing operational efficiency.
Conclusion
Harnessing edge computing to scale AI-enabled applications represents a significant leap toward realizing the full potential of artificial intelligence. By addressing latency, bandwidth, security, and scalability challenges edge computing provides a robust infrastructure for deploying AI solutions across various industries. FusionLayer’s innovations and expertise play a crucial role in this landscape, ensuring reliable and efficient network connectivity essential for edge computing.
As edge computing technology continues to evolve, it will undoubtedly play an integral role in shaping the future of AI, driving innovation, and transforming how we interact with the world around us. The synergy between edge computing and AI promises not only to enhance current applications. but also to unlock new possibilities that were previously unimaginable. With FusionLayer at the forefront of this technological evolution, the future of AI at the edge looks incredibly promising.
Reply a Comment